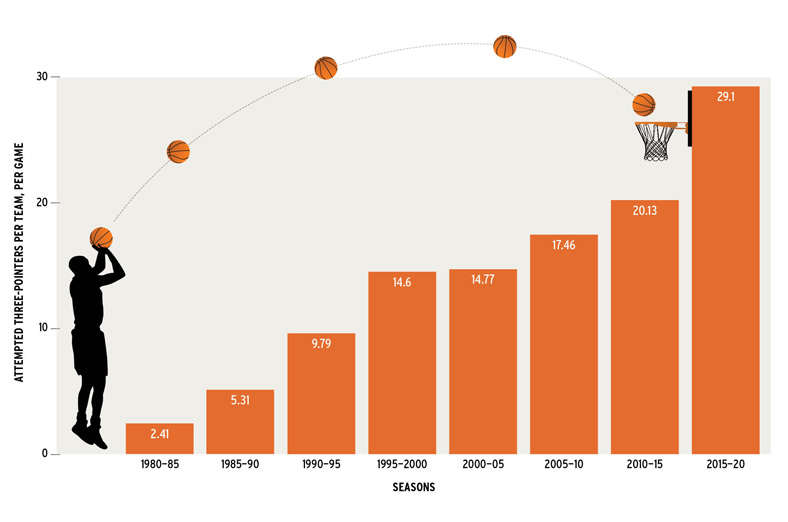
In 2016, Grant Fiddyment was drafted by the Philadelphia 76ers—as a data scientist.
The Georgia native, who grew up during the Atlanta Hawks’ Dikembe Mutombo era, was a slam dunk hire for the 75-year-old franchise’s research and development team. The AU professorial lecturer is now a manager in the department, which boasts a roster of five data wonks.
“I enjoy building things and solving puzzles, so that makes programming a fun job already,” says Fiddyment, who analyzed the Celtics to practice programming and machine learning techniques as a doctoral student in computational neuroscience at Boston University. “The fact that I can apply it all to basketball is like the cherry on top.”
The sweet part of NBA data modeling—a field that sparked several league-wide shifts (see above)—is that it’s applicable year-round. In-season, Fiddyment’s team largely focuses on game work, utilizing data collected with video tracking software to analyze plays, lineup combinations, outcomes, and more for the coaching and scouting staffs. Breaking down 82 games—72 this season, which started in December—in less than eight months might seem like a grind, but it moves about as quickly as a 24-second shot clock.
“A new season is both very exciting and very busy at the same time,” says Fiddyment, who teaches predictive analytics and Python programming courses at the Kogod School of Business. “Then suddenly, it feels like a day later the playoffs are starting.”
During the offseason, his work shifts to preparing for make-or-break roster-building windows like the NBA draft.
For his data team’s work to have a positive impact, it must translate from code to court. Fiddyment’s mentors at BU—mathematical science professor Mark Kramer and statistics professor Uri Eden, with whom he worked to develop simulations of epileptic seizures—instilled in him the value of simple models.
“While we always have to strike a balance between simple and realistic models, the [former] becomes really important when we have to explain the model and its predictions to someone else,” he says.
In basketball, as in brain analysis, “You need to know exactly how the data are being collected and processed” and think carefully about how to measure them—and their limits—Fiddyment says.
A good data scientist, much like a guard with ice-cold veins and a red-hot shooting hand in the fourth quarter, knows how to filter out the noise.
THREE'S a party
One of the great data revolutions in the NBA has been the explosion of three-point shooting. Since the rule change adding the 23-foot, 9-inch arc prior to the 1979–80 regular season, the number of per team, per game three-point attempts has increased tenfold.
1980–85: 2.41
1985–90: 5.31
1990–95: 9.79
1995–00: 14.6
2000–05: 14.77
2005–10: 17.46
2010–15: 20.13
2015–20: 29.1