Data Science Practicum
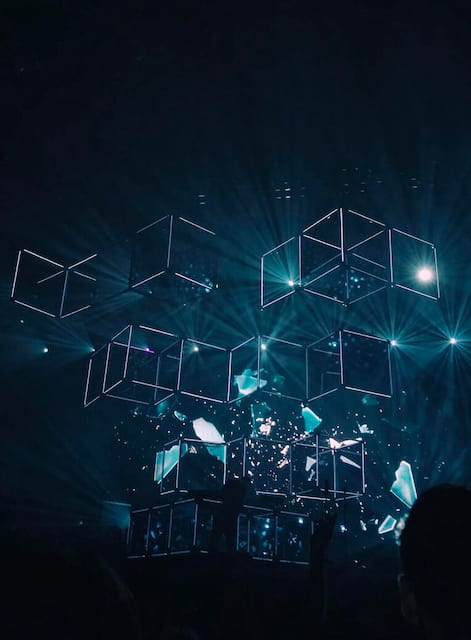
The Data Science Practicum (DATA-793) is the capstone experience for the MS in Data Science and provides assistance to faculty, government organizations, and companies. See examples completed Student Projects.
Students entering the practicum have completed coursework in statistics, regression, and R for data science, along with completed or ongoing coursework in statistical machine learning. Practicum students are ready to put their visualization, analytics, and data modelling skills to work on live projects.
Practicum Coordinator:
Maria Barouti, barouti@american.edu.
Call for Projects: Faculty & Staff
Let our advanced students help with your data:
- Use the Faculty Project form to provide your project title, description, AU email, and required skills — please use our course listings to help specificy required student skills or classes.
- Under the guidance of our faculty, students in the Data Science Practicum (DATA-793) or other advanced research courses review available projects for best fits and contact you.
- You and the student(s) agree on a plan for work on the project.
Current Projects Now Inviting Student Participation
Please browse descriptions further below for details on each project:
- Networks of Influence and Support Between War and Peace
- Addressing Fairness Issues in Imbalance Datasets
- Growth Spurts: Fact or Fiction?
- Improving Access to Electrical Power for Climate Resilience TOP 23F Sprint
- Predicting Clinical Trial Progression based no Trial Descriptions using pre-trained machine learning models
- Rural/Urban Differences in Age-Adjusted Incidence Rates of Pediatric Cancer in the United States, 2000 -2019 (SEER data)
- Unmasking Antisemitism
- Predicting Failure of Vehicle Components
- Tackling the Problem of Learning Long-Tailed Distributions with Error Correcting Codes
- Analysis of State Teacher Licensure Exam Pass Rates
- Support Teacher Diversity Strategy Development through Predictive Analytics and Real-Time Teacher Retention Dashboards
- Text Mining on Big Data
- Metadata Preprocessing and Information Extraction
- Annotation Tool Selection
- Survey Data from Online Psychology Experiments
- A Comparative Study of Litigation Data Related to India’s Agricultural Land Laws
- The Adopt a Pixel Project
- Online Antisemitism Detection Using Multimodal Learning
- Exploring Multi-Task Motor Learning
- Detecting Storytelling Tactics in Text Using Machine Learning
- Statistical Consulting Class: Stat 798
- Lh Dynamics in African and Asian Elephants
- Characterizing Temporal Patterns in Longitudinal Prolactin Secretion in African Elephants
- Understanding the Effect of Deep Architectures on the Class Imbalance Problem
- Searching for Signatures of Elusive Stellar Coronal Mass Ejections from Young Suns Using X-Ray Data
- Estimating Physical Properties from Videos with Neural Networks
- Inclusion by Design
- Application of Machine Learning on the Survival Analysis of Breast Cancer Patients
- The Accountability Project
- Trustworthy Machine Learning
- Data-Driven Material Estimation from Images
Networks of Influence and Support Between War and Peace
Hundreds of international actors work in fragile and conflict-affected countries to build peace, prevent violent extremism, reduce poverty, save lives, or rebuild infrastructure. They are connected to each other and to state and non-state actors through formal contracts, informal relationships, and regular coordination meetings. This project studies these networks of influence and support and their effect on indicators of conflict and peace. See more at https://www.ripilab.com/blog/creatingnoidatasets/.
Prerequisites: data wrangling, regression, and R
Contact: Susanna Campbell, susanna.campbell@american.edu
Addressing Fairness Issues in Imbalance Datasets
Addressing bias issues in imbalanced datasets, like credit card fraud detection, is crucial for developing fair and accurate machine learning models. One approach to mitigating bias involves leveraging Universum data in Support Vector Machine (SVM) models. In this project, we will incorporate Universum data into the training process so that SVM models can learn a more nuanced decision boundary that better generalizes across both classes, reducing the impact of bias. Students interested in this project would implement several related SVM models to interesting applications like disease detection where number of positive results are significantly lower than negative results.
Prerequisites: Stat 627, Data 612, Data 613
Contact: Ahmad Mousavi, mousavi@american.edu
Growth Spurts: Fact or Fiction?
Are growth spurts in children a figment of our imagination? CDC growth charts all show concave down height curves from birth until adult age. This does not preclude that individuals may experience growth spurts, but do they? Often, we say that kids hit growth spurts around puberty, however, we also know that adult hormones actually suppress growth, which is why puberty blockers are given for precocious puberty, to prevent premature growth stabilization. An alternate theory is that as children grow, as they begin to reach adult eye-level, adults perceive a faster angular change in the child's height. Also, noise in data collection may lead to false assumptions about the existence of growth spurts.
If this project is selected, the student would find appropriate data sets to research this question and answer it, including hypothesizing why people may be claiming that children experience growth spurts, if this is not truly occurring.
Prerequisites Requirements would depend on the data sets located by the student.
Contact: Donna Dietz, dietz@american.edu
Predicting Clinical Trial Progression Based on Trial Descriptions Using Pre-trained Machine Learning Models
In this NSF-funded project, we aim to understand what factors contribute to progression of a clinical trial from Phase 1 to 2. We have access to clinical trial data and trial descriptions. We use pre-tranied medical text language models (e.g. BERT and Transformers) to see if we can predict new trials's outcome. The student is expected to take a machine learning and/or NLP courses.
Prerequisites Python, Numpy, PytTorch, Pandas, Git and GitHub, Statistics, Have courses in machine learning
Contact: Bei Xiao, bxiao@american.edu
Rural/Urban Differences in Age-Adjusted Incidence Rates of Pediatric Cancer in the United States, 2000-2019 (SEER data)
Capstone project-MPH-Epidemiology. Specific Aims:
- To examine rural-urban differences in the time trend of age-adjusted incidence rates of pediatric cancer in the United States from 2000-2019.
- Hypothesis: The incidence of pediatric cancer in the U.S. from 2000- 2019 is higher in rural compared to urban populations.
- To investigate whether socioeconomic status (SES) is a modifier in the relationship between rural/urban population and incidence of pediatric cancer.
Prerequisites Have taken all core courses for Epidemiology, however SEER is new. Need to extract data from SEER, do Join point analysis which is again new.
Contact: Maddy P, madhurp87@gmail.com
Unmasking Antisemitism
This project studies coded antisemitic hate speech in extremist and mainstream social media outlets. The project started with a collection of seed words known to be antisemitic. Data was scraped off barely moderated extremist social media platforms like 4Chan and Truth Social and then analyzed. To track the spread of extremist antisemitic language to the general population, the requested work for a semester-long effort would scrape videos from YouTube and examine them for antisemitic hate speech. Data analysts would gather the videos and determine the best storage method through discussion with a faculty mentor. The videos would then be coded for antisemitic language and images based on an established coding statement. The work will become part of a larger antisemitic project conducted by cross disciplinary faculty members as part of American University’s Signature Research Initiative, which is sponsored by the Provost’s Office of Research.
Prerequisites MS in Data Science Student
Contact: Jeff Gill, Director of the Center for Data Science, jgill@american.edu.
Predicting Failure of Vehicle Components
Condition-based maintenance plus (CBM+) is a strategy that monitors the real-time conditions of a vehicle in operation to determine what maintenance needs to be performed and predict future maintenance or failure points. This differs from typical preventative maintenance strategies in that it is based off real-time data, as opposed to calendar-based or mileage strategies. The purpose of this project is to analyze and explore Controller Area Network (CAN) data from multiple vehicles in order to develop predictive algorithms to begin to provide the Remaining Useful Life (RUL) of the different platforms/vehicles.
Students will develop models that follow a data-driven approach as opposed to the standard physics-based approach, which focuses on exploiting known relationships between sensor signals. Students will work with anonymized data to identify signals of interest, normal patterns of operation, and deviations/abnormalities from those patterns indicating maintenance is due soon.
Prerequisites US Citizenship, Knowledge of Python and Common Packages (numpy, pandas, scikit-learn, scipy, etc.), an interest in finding value in seemingly random data patterns
Contact: Connor Cabrey, connor.cabrey@tridsys.com
Tackling the Problem of Learning Long-Tailed Distributions with Error Correcting Codes
In classification problems, long-tailed distributions correspond to multiclass problems for which some classes are represented by large numbers of samples, while others are represented by only a few. It is, in some sense, an extension of the classical class imbalance problem. A traditional ensemble method used to enhance the performance of multi-class classifiers uses Error Correcting Codes and was previously used to tackle class imbalanced situations. The purpose of this project is to investigate whether this approach is competitive in Deep Learning long-tailed problems.
Prerequisites Experience with Deep Learning models applied to image data; Good programming skills in Python
Contact: Nathalie Japkowicz, japkowic@american.edu
Analysis of State Teacher Licensure Exam Pass Rates
Our program participants are required to pass a set of teacher licensure exams for entry and completion of our educator preparation program. The timing and type of exam required varies by state and by licensure area, with these state requirements dynamically changing over the years. Urban Teachers collects licensure exam data from two external testing vendors and uploads into our internal data platform. We are interested in: 1) an initial quality analysis and cleaning of our data, 2) an analysis of the number of attempts and pass rates, in order to understand if there are trends by participant demographics and type of exam, 3) the financial costs to our participants, and 3) the development of a report or dashboard that ensures alignment between participant’s program of study, licensure area, clinical placement (grade/subject), and exams taken. This work can inform programmatic decisions on where to devote specific resources and supports as well as internal policy decisions on testing requirements.
Prerequisites Knowledge of R and willingness to learn Power BI for the development of a dashboard
Contact: Viticia Thames, viticia.thames@urbanteachers.org
Support Teacher Diversity Strategy Development through Predictive Analytics and Real-Time Teacher Retention Dashboards
Teacher attrition from our teacher preparation program can happen either because a teacher candidate was dismissed, or because the teacher candidate resigned from the program. We would like to conduct predictive analytics for reasons behind these program exits to determine whether there are trends by reasons for dismissals and resignations that vary by teacher candidate demographic background. And we would like insights from predictive analytics to be used to inform the creation of Teacher Retention Dashboards at each of our sites: DC, Baltimore, Dallas/Fort Worth and Philadelphia. Insights from this project will generate data-informed strategies that can increase program teacher retention across all four of our teacher preparation sites.
Prerequisites Experienced with R, covariance analysis by teacher demographic
Contact: Viticia Thames, viticia.thames@urbanteachers.or
Text mining on big data
Identifying entities of interest in text is an important precursor for many data mining, natural language processing and natural language understanding tasks. We are interested in processing large English text corpora to recognize text strings that denote named entities of interest such as diseases, symptoms, and laboratory measurements as well as personal information such as age, gender and occupations of patients.
Students will develop algorithmic solutions to recognize a particular set of named entities in a large corpus of text. A relevant set of metadata will be provided. Students can write their own algorithms or use an off-the-shelf tool of their choice to accomplish the task. If any training dataset is available for that particular task, a supervised machine learning approach can be taken, but the students might need to extend the size of the training data to increase the system’s predictive performance.
Prerequisites Students must be competent in at least one programming language, preferably C, C++, Perl, or Python. Additional requirements are a good mathematical background, some background in natural language processing and machine learning methods.
Contact: Dr. Mehmet Kayaalp, mkayaalp@mail.nih.gov
Metadata preprocessing and information extraction
To understand the content of any text, we need to identify entities that are represented in words and phrases. Resources that contain such entities in a semi-structured fashion are very helpful. The target audience of such a resource is usually either a human or a specific application. Some of these resources are not curated professionally but produced by volunteers, making the end-product less coherent and noisy.
At NIH, we work on several metadata sets, which need to be preprocessed to make the metadata more coherent so that we can read them algorithmically and extract necessary information reliably. Students may choose to work either on biomedical metadata such as vocabularies in UMLS or on general metadata such as Wikidata along with text corpora such as PubMed Central and Wikipedia. Through this practicum, students will be working on big data, identifying and correcting errors, and transforming the data into a suitable format so that they can be digested by in-house applications.
Prerequisites Students must be able to write programs to perform text processing and be competent in at least one programming language, preferably C, C++, Perl, or Python. Some background in natural language processing would be very valuable.
Contact: Dr. Mehmet Kayaalp, mkayaalp@mail.nih.gov
Annotation tool selection
The success of modern artificial intelligence (AI) systems partly depends on the availability of large annotated / labeled datasets. They are essential not only to train such systems but also to evaluate their output.
At NIH we build freely available AI applications using such annotated datasets. Unfortunately, our annotation tool is not easy to use for our users. We need to identify a capable annotation tool that is easy to use and available to our users free of charge.
There are a number of such annotation tools on the market. The students will be tasked to learn the annotation requirements of the on-going project at NIH, search through all available tools, evaluate them based on the requirements of the project, and select the most likely candidates.
Prerequisites Students should be able to install and test annotation applications on their own. Access to a Windows or a Linux computer.
Contact: Dr. Mehmet Kayaalp, mkayaalp@mail.nih.gov
Survey data from online psychology experiments
Mood disorders, such as depression, are a major cause of suffering worldwide. In order to improve scientific understanding of mood, our clinical research colleagues ran a series of experiments where they asked participants to continually rate their mood while playing a simple gambling game online. Participants were also asked to respond to a survey eliciting their daily happiness, ability to enjoy life, and their experience of the gambling game. From these surveys and the logs of the gambling game, we would like to explore possible associations between survey responses and mood dynamics during the gambling game, which could be of relevance to our scientific understanding of the causes or effects of mood. Students working in this project will gain experience in processing data collected from online surveys, visualization and analysis of survey data, and in formulating and testing statistical hypotheses.
Prerequisites Statistics and/or machine learning; knowledge of python (with numpy and pandas) or R
Contact: Charles Zheng, charles.zheng@nih.gov
A Comparative Study of Litigation Data related to India’s Agricultural Land Laws
Agricultural land in India is heavily regulated. It is a state subject. State laws regulate who can be a farmer, what a farmer can do with the land, whom a farmer can sell the land to, how much land a farmer can own, and under what conditions the State can compel acquisition of farm land. Details vary from state to state. The students working on this project will work to web scrape the judgments/ case filing data related to agricultural land laws from the central case law repository and undertake a comparative frequency analysis for various India states. The goal of this project is to explore how litigation frequency has varied both, over time and with the kind / degree of restrictions on agricultural land and to identify the most contested/ litigated issues with respect to agricultural land. Students will apply a range of statistical and data science techniques, including web scraping, data tidying, visualization, and data exploration to obtain and analyze legal case data.
Prerequisites Knowledge of web scraping techniques is essential. In addition, knowledge or R (or Python) for data tidying, visualization and exploration; Statistics.
Contact: Prashant Narang (CCS, India) & Dr. Nimai Mehta (Math & Stat Department, AU), mehta@american.edu
The Adopt a Pixel Project
The Adopt a Pixel Project needs data scientists to build tools that will extract NASA satellite image areas and corresponding citizen science ground photos, and then associate them in a digital platform for analysis by citizen scientists. The project employs the high profile Zooniverse citizen science platform.
Students will implement strategies and methods to automate this extraction, processing and publishing of the data on the Zooniverse platform. The analyzed data will be used for further analysis and visualization in data dashboards, websites and programmatic notebooks. The resulting data stream will support scientific tasks such as graphing, mapping and data reduction and AI.
Contact: Peder Nelson, Oregon State University, College of Earth Ocean and Atmospheric sciences. Peder is an instructor of geography and geospatial sciences, and the NASA GLOBE Observer Land Cover Science Lead. peder.v.nelson@gmail.com
Online Antisemitism Detection Using Multimodal Learning
Increasing cases of online antisemitism have become a major concern due to its socio-political consequences. The task of detection of online antisemitism poses multiple challenges that include the extraction of joint representations from multi-modal data (e.g. text, images, etc). Students working on this project will work with different data fusion approaches based on latent variable analysis and deep neural networks in order to extract joint representations from multi-modal data so that online detection of antisemitism and knowledge discovery are achieved jointly.
Prerequisites: Machine Learning, good knowledge of Python
Contact: Nathalie Japkowicz and Zois Boukouvalas, boukouva@american.edu
Exploring multi-task motor learning
How does our brain allow us to learn countless complex motor skills, from tying our shoes to hitting a tennis serve? How and why do previously learned skills affect how well we learn a new skill, and are such interactions reflected on the neuronal and memory level? Answers to these questions are not only important for basic neuroscience, but also for education, rehabilitation and the improvement of artificial neural networks for multi-task learning. We train rats in a high-throughput manner on multiple motor skills and track their performance and fine-grained movement trajectories over tens of thousands of trials. The goal of this project is to explore how learning of multiple motor skills differs from learning individual skills and how skills interact on the behavioral level under various training conditions. Students will explore our large performance and movement datasets to determine the relationship between learned skills and how it develops over the course of training.
Prerequisites Matlab and/or Python
Contact: Steffen Wolff, swolff@som.umaryland.edu
Detecting Storytelling Tactics in Text Using Machine Learning
Research in entertainment theory has shown that people are more effectively mobilized through engagement and entertainment than through overt and explicit persuasive arguments. Utilizing entertainment tactics - or storytelling tactics - has been shown to “transport” readers into the story world, ultimately leading to more effective communication campaigns. For this effort, Protagonist is interested in building a machine learning classifier to better identify the storytelling potential of media articles and social media campaigns. Protagonist will provide a list of storytelling features known to contribute to a “state of transportation” and practicum participants will create a model to classify text data as “transportive” based on the presence of those features in multilingual text-based data. Practicum students may also employ feature selection techniques to identify the strongest predictors of transportation in text data.
Prerequisites Knowledge of machine learning, text mining, and Python
Contact: Becky Owens, Maria Barouti, bowens@protagonist.io
Statistical Consulting class: Stat 798
In this class, you will work with a statistics professor to learn new techniques, such as:
1) Determination of sample sizes and randomization of subjects to include in an experiment or survey
2) Design of a survey sampling and optimal experiments
3) Choice of the proper statistical methods for studies and experiments
4) Transformation and import of data into desired statistical software packages
5) Interpretation of statistical analysis results for researchers
6) Assessment of the power of statistical tests (power analysis)
7) Software support for major statistical software packages: R, SPSS, SAS, STATA, etc.
8) Assistance with the statistics section of a research manuscript before submission to a journal
Permission of the Director of Data Science (malloy@american.edu) and the Director of the Statistical Consulting Center (kapatou@american.edu) is required.
Prerequisites If you have a strong statistical background, and 2)you would like to work on a variety of short and long projects then you can join STAT 798!
Contact: Aleka Kapatou, kapatou@american.edu
LH Dynamics in African and Asian Elephants
Both Asian and African elephants exhibit a unique hormone pattern during the follicular phase of the estrous cycle with two luteinizing hormone surges that occur approximately 3 weeks apart (double LH surge); the surges are indistinguishable, but only the second one induces ovulation. The goal of this project is to summarize decades of hormonal data and identify intra- and inter-elephant differences in various aspects of the double LH surge: time from the decline in progestagens during the luteal phase to the first LH surge; time between LH1 and LH2, time from LH2 to the rise in progestagens after LH2-induced ovulation. Some elephants have even demonstrated three surges during the follicular phase, which also need to be documented and compared to the normal double LH surge.
Prerequisites Data manipulation and visualization, knowledge of R.
Contact: Natalia Prado, nprado@adelphi.edu
Characterizing Temporal Patterns in Longitudinal Prolactin Secretion in African Elephants
Normal cycling African elephant females have temporal patterns in prolactin secretion, while acyclic females with abnormal prolactin levels (too high or low) appear to lose this temporal pattern. The goals of this project are to describe normal temporal prolactin patterns in elephants and determine how acyclic females change or lose their prolactin temporal patterns. In doing so, we aim to better understand underlying causes for hyperprolactinemia, a reproductive disorder that is associated with ovarian acyclicity in African elephant females.
Prerequisites Data manipulation and visualization, knowledge of R.
Contact: Natalia Prado, nprado@adelphi.edu
Understanding the effect of Deep Architectures on the Class Imbalance Problem
The purpose of this project is to study the behavior of deep learning systems in settings that have previously been deemed challenging to classical machine learning systems to find out whether the depth of the systems is an asset in such settings.
Prerequisites CSC-680, Python, Scikit-Learn, TensorFlow Keras, Colab
Contact: Nathalie Japkowicz, japkowic@american.edu
Searching for Signatures of Elusive Stellar Coronal Mass Ejections from Young Suns Using X-ray Data
NASA’s Kepler and TESS missions have revealed frequent explosive events called superflares from many planet hosting dwarf stars, providing a mechanism by which host stars may have profound effects on the physical and chemical evolution of exoplanetary atmospheres. Solar studies suggest that large flares are accompanied by fast ejection of coronal magnetized materials referred to as coronal mass ejections or CMEs. However, astronomers do not have reliable methods to detect and reveal these elusive stellar magnetic ejections from available data. My team and scientists from Penn State have collected large sets of data based on Chandra and XMM-Newton X-ray observations on hundreds of explosive events from very young stars resembling our Sun in its infancy. In this project, students will explore how statistical and machine learning techniques can be used to search for signatures of elusive CMEs. Students do not need to have any background in astronomy.
Prerequisites Statistics, Machine Learning, knowledge of Python, or IDL/Matlab.
Contact: Vladimir Airapetian, vladimir.airapetian@nasa.gov
Estimating physical properties from videos with neural networks
Accurately estimating physical properties of objects from visual and multimedia inputs (e.g. stiffness, roughness, softness) is important for automatic scene understanding in everyday tasks in an AI system. This project aims to leverage human knowledge and physics to learn to estimate physical properties of objects in image/video using deep learning models, with a special emphasis on learning from limited data and with built-in uncertainty in the model.
Prerequisites:Numpy/Scipy/Python, Web programming, Basic machine learning, Linear Algebra, CSC476 Computer Vision is a plus.
Contact Bei Xiao, bixao@american.edu.
Inclusion by Design
Minority inclusion is at the center of not only creating more equal societies but also democratic stability and preventing ethnic conflict. Yet scholars have found no solution to the knotty problem of measuring inclusion across countries. This problem limits our ability to learn how to design political institutions, such as the electoral system or federalism, to enhance minority inclusion more effectively. My solution to this problem centers on estimating minority electoral support for governing parties in legislatures (and for winning presidential candidates where the president serves more than a symbolic role). Using this information, one can also estimate the minority share of the government’s (and the president’s) electoral supporters. Towards that end, I am taking a multipronged approach to estimating voting behavior by different groups, relying on both ecological inference and polling data.
Prerequisites I need students who are very comfortable (1) locating polling data, and (2) getting key descriptive stats properly weighted out it. Appropriate skills in statistical packages are helpful. I have a grant to pay students over the summer who are interested.
Contact: David Lublin, dlublin@american.edu.
Application of Machine Learning on the Survival Analysis of Breast Cancer Patients
This project is an attempt to study the applications of machine learning techniques in Weka (Clustering, Classification, Association rules, regression) for the survival analysis of breast cancer patients. The National Cancer Institute’s Surveillance, Epidemiology, and End Results (SEER) Public-Use Data (years 1977-2017) breast cancer data set will be used for all experiments and R programming for visualization. The aim of this project is to investigate the significance of the prognostic factors such as age, ethnicity/race, tumor grade, tumor size, etc. on the survival of breast cancer patients.
Prerequisites Knowledge of R, Machine Learning Algorithms, Weka
Contact: Mehdi Owrang, owrang@american.edu.
The Accountability Project
The Accountability Project was built as a tool to help researchers and newsrooms search across otherwise siloed data. We acquire, process and standardize hundreds of data sets, accounting for nearly 900 million records. We are seeking student researchers who are interested in either contributing data to the project or analyzing data within the project.
Prerequisites We are software agnostic, but it requires skills in basic data review, cleaning and analysis. Statistics not required, but rigorous data standards are. We're especially interested in folks who want to tell stories with data.Most of the team uses either R or SQL.
Contact: Jennifer LaFleur, lafleur@american.edu.
Trustworthy Machine Learning
The deployment of machine learning in real-world systems is growing faster than many had predicted. Today, organizations across different industries are increasingly using machine learning to augment human decision making, reduce costs and enhance productivity. Recent research has shown that malicious actors can use modified input data to make a machine learning algorithm behave in unexpected ways. For example, researchers have shown that they can trick the machine learning based computer vision algorithms designed for self-driving cars to mistake stop signs for speed limit signs. Thus, it calls for technologies that will ensure that machine learning is trustworthy so we rely on them to produce reliable outputs. Students working this project will study and investigate the trustworthiness of machine learning models.
Prerequisites Already have a basic understanding of machine learning. Python required. Computer vision is a plus.
Contact: Leah Ding, ding@american.edu
Data-driven material estimation from images
We are interested in estimating 3D shape, material attributes and classes from photographs of translucent materials. The project involves assisting the PI to a large image dataset of translucent objects and use unsupervised learning and representational learning techniques to learn image features that are useful for teasing apart causal factors (geometry, lighting and optical properties) that influencing material properties from images. In addition, the project requires building crowd-sourcing experiments to collect human annotation from online-platform such as Amazon Mechanical Turks and compare the data with outputs from the machine learning models.
Prerequisites Python(Numpy, PyTorch), Basic Machine Learning, Deep Learning, Statistics.
Contact: Bei Xiao, bxiao@american.edu
Top image credit: Fabio, Unsplash.